Unlike humans, robotaxis are unable to solve a problem they have never encountered before
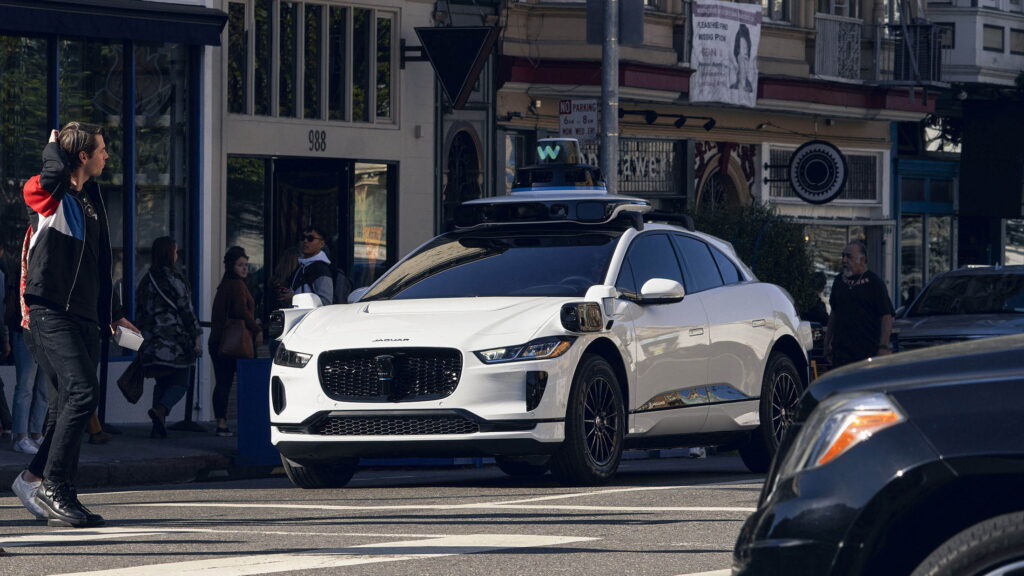
The development of autonomous vehicles has taken much longer than many in the industry predicted. One of the main factors slowing progress is the fact that the artificial intelligence currently used in vehicles cannot connect cause to effect.
While AI is impressive, it is not intelligent in the way we consider a human. The technology is currently not allowed to reason or distract, at least not in most vehicles. This means that robotaxis is unable to solve problems when confronted with a new situation.
As a result, the chaos of the real world can be a major problem for AVs, Autonews reports. The industry calls strange, new scenarios “edge cases,” and that’s the term former Cruise AV CEO Kyle Vogt used to describe the incident that took the company’s robot axle off the road last year.
In that circumstance, a woman was hit by another vehicle and bounced off Cruise’s autonomous vehicle. I would hazard a guess that the vast majority of us have never experienced that, but we would all know to stop, rather than try to pull over, as the robotaxi did, leaving the injured woman a few feet away. was dragged away. It’s not that the car was evil, it just couldn’t tell what was going on and wasn’t gifted with the ability to predict what impact its actions might have.
Read: Cruise recalls Robotaxis for a software solution to stop them from dragging pedestrians across the road
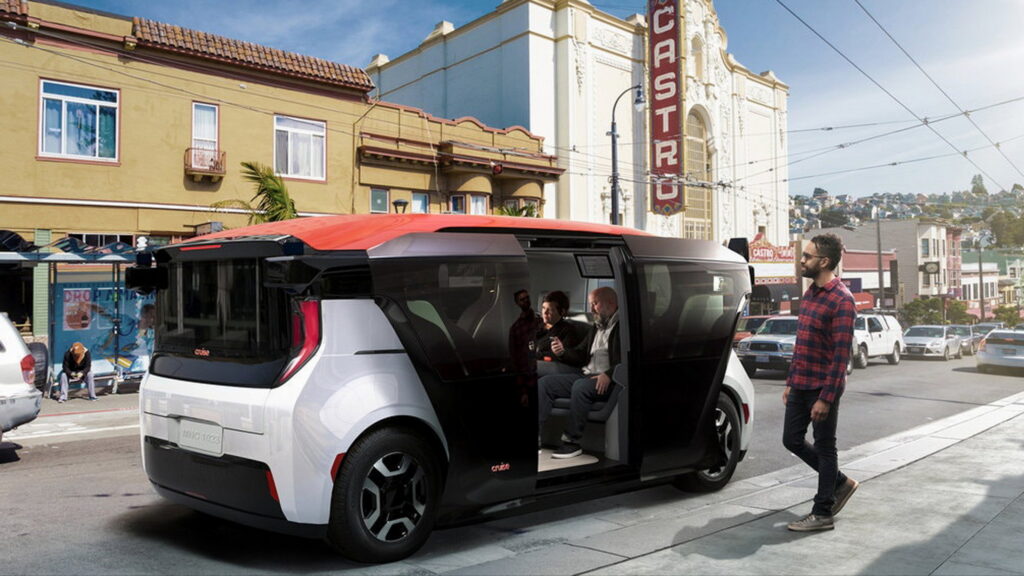
However, autonomous researchers do have some strategies to avoid making bad decisions. For example, human operators can take over in situations where the program is not prepared. Data from those incidents is monitored, fed back into simulators, and the human response is used to try to train the vehicle for the future.
Training challenges and limitations
Furthermore, some companies simply try to think of as many scenarios as possible before the vehicle even hits the road. This could involve manual coding or the staging scenarios used to train autonomous vehicles in a safe place. While researchers admit they can never figure out every edge case, this solves at least some of them.
However, some experts now believe that these training methods may never effectively prepare a robotaxi for the chaos of the real world. Without causal reasoning, AI may never be able to navigate all edge cases.
“The first time he sees something different from what he was trained for, does someone die?” Phil Koopman, an IEEE senior member and professor at Carnegie Mellon University, told Autonews. “The whole machine learning approach is reactive to things that have gone wrong.”
Solving the problem means more than just giving AI the ability to reason causally. In fact, the technology has so far been prevented from making too many causal judgments to prevent unpredictable false positives from occurring. That was a decision made for safety reasons, but it leaves researchers with a paradox to solve if they want to create robotaxis that can truly navigate roads autonomously: should AVs make mistakes because they are stupid, or because they went the wrong way? conclusion?
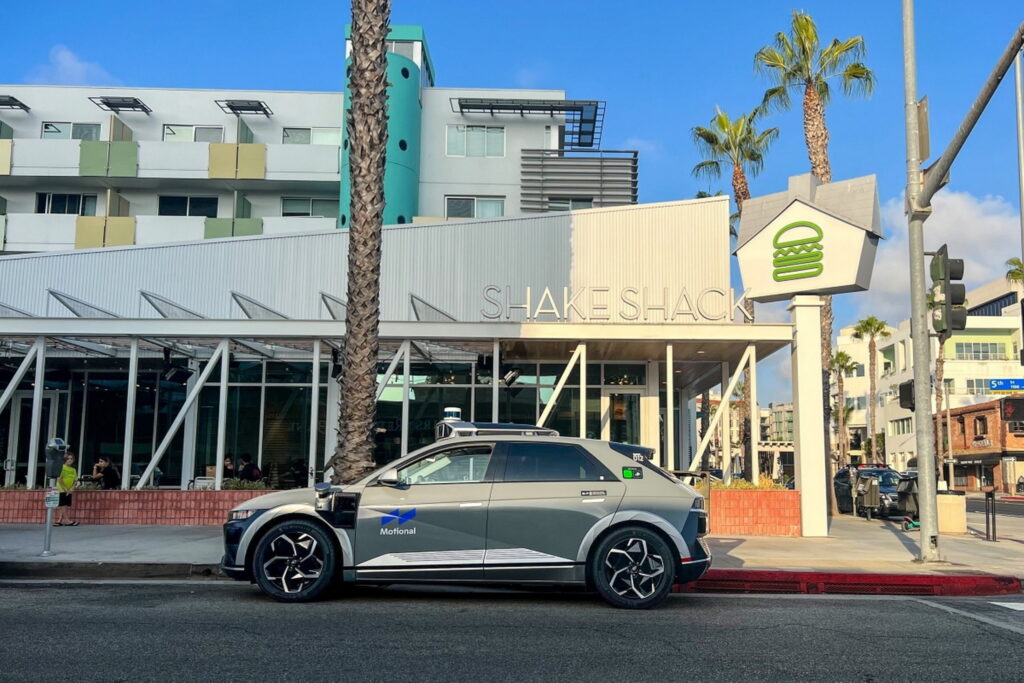